Correlation Analysis: Unlocking The Secrets Behind Data Relationships
Hey there, data enthusiasts! If you've ever been curious about how variables interact and influence each other, you're in the right place. Correlation analysis is like the secret decoder ring of data science. It helps us understand the relationships between different variables, and trust me, it's way more exciting than it sounds. Whether you're a business analyst, a student, or just someone fascinated by numbers, correlation analysis is your golden ticket to making sense of the world around you. So, let's dive in and uncover the magic behind this powerful statistical tool!
Now, before we get too deep into the nitty-gritty, let's clear something up. Correlation analysis isn't just for math nerds or people in lab coats. It's a practical tool that anyone can use to make better decisions. Imagine you're running a business and you want to know if increasing your marketing budget will actually lead to more sales. Or maybe you're a health enthusiast trying to figure out if eating more vegetables really improves your mood. Correlation analysis can help answer those questions and so much more.
But here's the deal: correlation doesn't always mean causation. We'll talk more about that later, but for now, just know that while correlation analysis shows us connections between variables, it doesn't necessarily prove that one thing causes another. Still, it's a powerful starting point for exploring data relationships and making informed decisions. So, buckle up because we're about to embark on a data-driven adventure!
Read also:Why Allsaints Popup Soho Is The Ultimate Fashion Destination
What Exactly is Correlation Analysis?
Let's break it down. Correlation analysis is a statistical method that measures the strength and direction of the relationship between two or more variables. Think of it like a ruler for relationships. It tells us how closely two things are connected and whether they move in the same direction or opposite directions. For example, if you're analyzing the relationship between hours spent studying and exam scores, you might find a positive correlation, meaning that as study time increases, exam scores tend to go up too.
There are different types of correlations, and understanding them is key to making sense of your data. The most common types are:
- Positive Correlation: When two variables move in the same direction. For instance, the more you exercise, the more calories you burn.
- Negative Correlation: When one variable increases while the other decreases. Think about the relationship between outdoor temperature and heater usage.
- No Correlation: When there's no apparent relationship between the variables. For example, the number of cups of coffee you drink might not have any connection to your shoe size.
Correlation is often represented by a correlation coefficient, which ranges from -1 to +1. A value close to +1 means a strong positive correlation, while a value close to -1 indicates a strong negative correlation. If the coefficient is near zero, it suggests little to no correlation.
Why Should You Care About Correlation Analysis?
Alright, here's the kicker: correlation analysis isn't just some fancy statistical tool; it's a game-changer for decision-making. Whether you're in business, healthcare, education, or any other field, understanding the relationships between variables can give you a competitive edge. For example:
In marketing, you can use correlation analysis to determine which advertising channels drive the most sales. In healthcare, it can help identify risk factors for diseases. In education, it can reveal the impact of different teaching methods on student performance. The possibilities are endless, and the insights you gain can lead to smarter strategies and better outcomes.
Biography of Correlation Analysis
Who Invented Correlation Analysis?
Correlation analysis has a rich history that dates back to the 19th century. Sir Francis Galton, a British scientist and cousin of Charles Darwin, is often credited with laying the groundwork for modern correlation analysis. He was fascinated by the idea of measuring relationships between variables and developed early methods for quantifying these relationships.
Read also:Pastel De Nata Blondies The Ultimate Sweet Fusion Thatrsquoll Make Your Taste Buds Dance
Later, Karl Pearson, another British mathematician, refined Galton's work and introduced the Pearson correlation coefficient, which remains one of the most widely used measures of correlation today. Thanks to these pioneers, we now have a robust framework for analyzing data relationships.
Name | Contribution | Year |
---|---|---|
Sir Francis Galton | Introduced the concept of correlation | 1880s |
Karl Pearson | Developed the Pearson correlation coefficient | 1890s |
How Does Correlation Analysis Work?
Now that you know what correlation analysis is and why it matters, let's talk about how it works. At its core, correlation analysis involves calculating a correlation coefficient, which quantifies the strength and direction of the relationship between variables. Here's a step-by-step breakdown:
Step 1: Collect your data. You'll need at least two variables to analyze. For example, you might collect data on advertising spend and sales revenue.
Step 2: Choose the right correlation method. The most common methods are Pearson's correlation, Spearman's rank correlation, and Kendall's tau. Each method has its own strengths and is suited to different types of data.
Step 3: Calculate the correlation coefficient. This involves some math, but don't worry—most statistical software can do the heavy lifting for you.
Step 4: Interpret the results. Look at the correlation coefficient to determine the strength and direction of the relationship. Remember, a value close to +1 means a strong positive correlation, while a value close to -1 indicates a strong negative correlation.
Common Misconceptions About Correlation Analysis
Before we move on, let's clear up some common misconceptions about correlation analysis:
- Correlation Doesn't Equal Causation: Just because two variables are correlated doesn't mean one causes the other. For example, ice cream sales and shark attacks might be correlated, but that doesn't mean eating ice cream causes shark attacks. There could be a third factor, like hot weather, influencing both.
- Not All Relationships Are Linear: Correlation analysis assumes a linear relationship between variables. If the relationship is nonlinear, you might need a different approach.
- Correlation Coefficient Alone Isn't Enough: While the correlation coefficient is a useful metric, it doesn't tell the whole story. You should also visualize your data using scatterplots to get a better understanding of the relationship.
Applications of Correlation Analysis
Correlation analysis is incredibly versatile and can be applied in a wide range of fields. Here are some real-world examples:
Business and Marketing
In the business world, correlation analysis is a go-to tool for understanding customer behavior and optimizing marketing strategies. For instance, companies can use correlation analysis to determine which product features are most closely linked to customer satisfaction or which advertising channels generate the highest return on investment.
Healthcare
In healthcare, correlation analysis helps researchers identify risk factors for diseases and evaluate the effectiveness of treatments. For example, studies might explore the correlation between lifestyle factors like diet and exercise and health outcomes like blood pressure or cholesterol levels.
Education
In education, correlation analysis can shed light on the factors that influence student performance. Researchers might investigate the correlation between class size and test scores or the relationship between parental involvement and academic achievement.
Tools for Conducting Correlation Analysis
Thankfully, you don't need to be a math wizard to perform correlation analysis. There are plenty of tools and software available to help you crunch the numbers. Some popular options include:
- Excel: A great starting point for beginners. Excel has built-in functions for calculating correlation coefficients.
- R and Python: If you're comfortable with programming, R and Python offer powerful libraries for conducting advanced correlation analysis.
- SPSS and SAS: These are industry-standard tools for statistical analysis and are widely used in academia and business.
No matter which tool you choose, the key is to understand the basics of correlation analysis and how to interpret the results.
Challenges and Limitations of Correlation Analysis
While correlation analysis is a powerful tool, it does have its limitations. Here are a few things to keep in mind:
- Outliers: Extreme values in your data can skew the results of your analysis. It's important to identify and handle outliers appropriately.
- Nonlinear Relationships: As mentioned earlier, correlation analysis assumes a linear relationship between variables. If the relationship is nonlinear, you might need to use a different approach.
- Sample Size: Correlation coefficients can be misleading if your sample size is too small. Always ensure you have enough data to draw meaningful conclusions.
Despite these challenges, correlation analysis remains a valuable tool in the data scientist's toolkit. By understanding its limitations, you can use it more effectively and avoid common pitfalls.
Best Practices for Correlation Analysis
To get the most out of correlation analysis, follow these best practices:
- Start with a Clear Question: Before you dive into the data, make sure you have a clear research question or hypothesis. This will guide your analysis and help you focus on the most relevant variables.
- Visualize Your Data: Scatterplots and other visualizations can provide valuable insights into the relationships between variables. Don't rely solely on correlation coefficients.
- Use Multiple Methods: Depending on your data, you might want to use multiple correlation methods to get a more complete picture of the relationships.
By following these best practices, you can ensure that your correlation analysis is accurate, reliable, and actionable.
Conclusion: Take Your Data Analysis to the Next Level
And there you have it, folks! Correlation analysis is a powerful tool that can help you unlock the secrets behind data relationships. Whether you're a business analyst, a student, or just someone fascinated by numbers, understanding correlation analysis can give you a competitive edge and help you make smarter decisions.
So, what are you waiting for? Dive into your data, explore the relationships between variables, and uncover insights that can drive success. And remember, while correlation doesn't always mean causation, it's a great starting point for exploring the world around you.
Before you go, I'd love to hear your thoughts. Have you used correlation analysis in your work or studies? What insights have you uncovered? Leave a comment below and let's keep the conversation going. And if you found this article helpful, don't forget to share it with your friends and colleagues. Happy analyzing!
Table of Contents
- What Exactly is Correlation Analysis?
- Why Should You Care About Correlation Analysis?
- Biography of Correlation Analysis
- How Does Correlation Analysis Work?
- Common Misconceptions About Correlation Analysis
- Applications of Correlation Analysis
- Tools for Conducting Correlation Analysis
- Challenges and Limitations of Correlation Analysis
- Best Practices for Correlation Analysis
- Conclusion

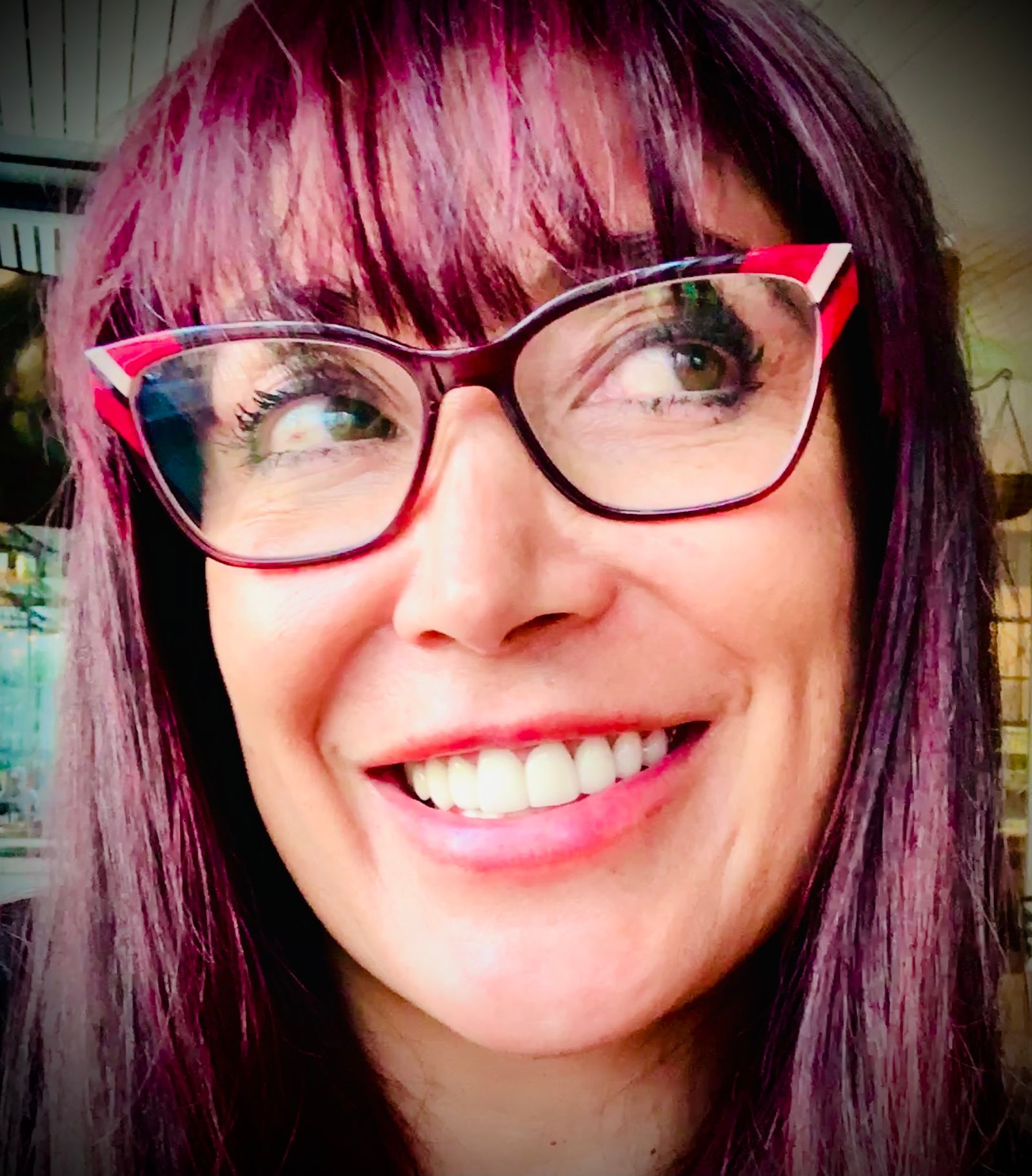
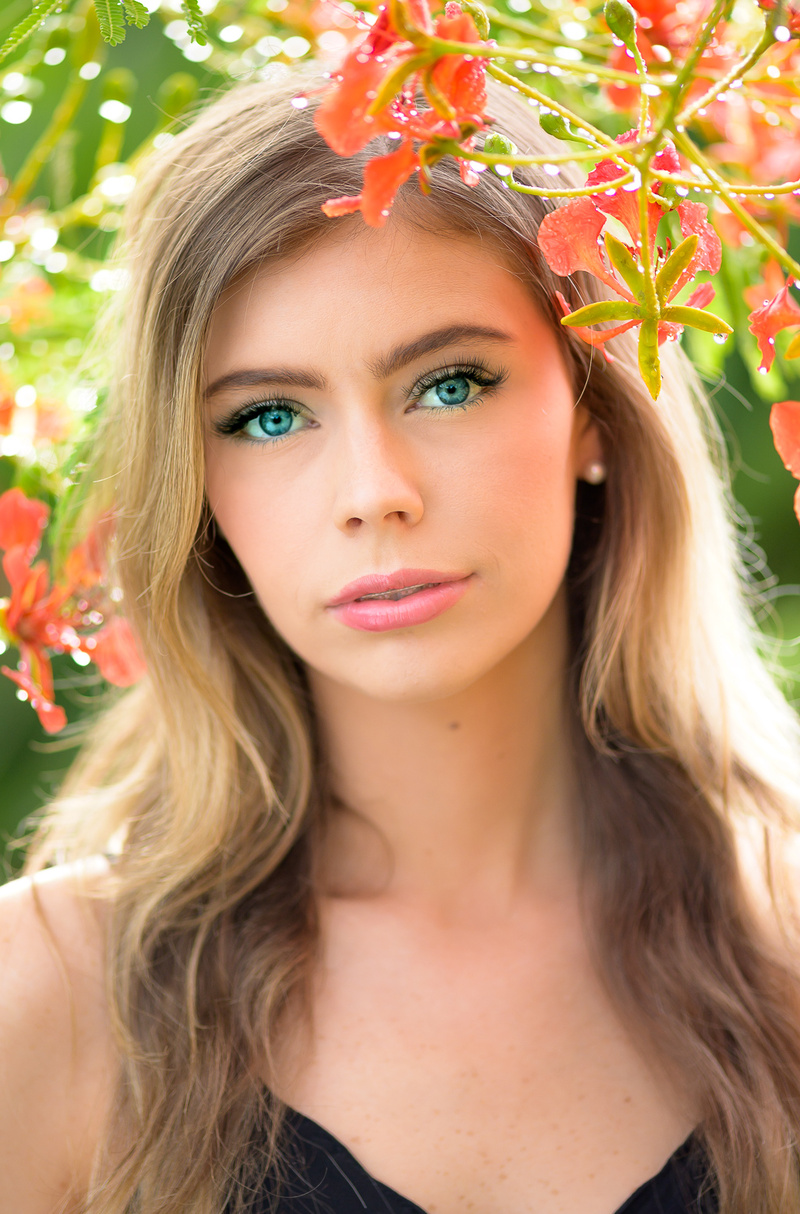